Fine-scale Surface Normal Estimation using a Single NIR Image
Youngjin Yoon* Gyeongmin Choe* Namil Kim Joon-young Lee In So Kweon
Korea Advanced Institute of Science and Technology (KAIST) Adobe Research
* The first and second authors have equal contributions on this work.
1
1
1
1
2
1
2
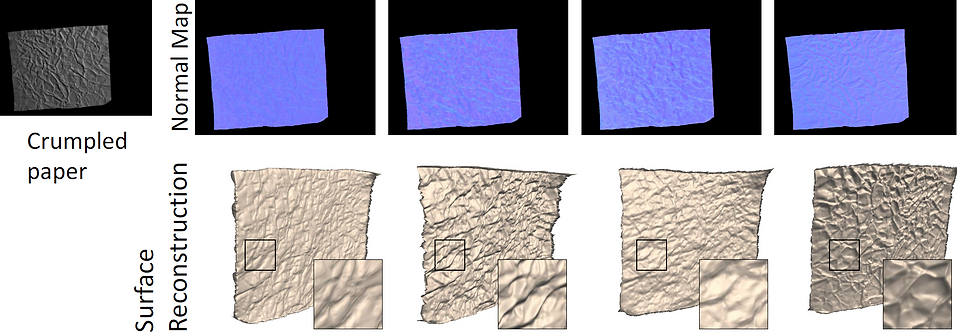
Input L2 L2 + Loss ang L2+Loss ang+Loss curl Ground-truth
Abstract
We present surface normal estimation using a single near infrared (NIR) image. We are focusing on .ne-scale surface geometry captured with an uncalibrated light source. To tackle this ill-posed problem,we adopt a generative adversarial network which is e.ective in recovering a sharp output, which is also essential for .ne-scale surface normal estimation. We incorporate angular error and integrability constraint into the objective function of the network to make estimated normals physically meaningful. We train and validate our network on a recent NIR dataset, and also evaluate the generality of our trained model by using new external datasets which are captured with a different camera under different nvironment.